Learn how insurance firms are utilizing AI and machine learning to detect and prevent fraud, saving billions. Learn how these technologies can combat insurance fraud.
Unprecedented amounts of fraud are occurring in the insurance industry, costing billions of dollars annually in false claims. Insurance companies are using machine learning (ML) and artificial intelligence (AI) to detect and stop fraud in order to combat this problem. In this post, we’ll examine the challenges and solutions associated with using artificial intelligence in the insurance industry to detect fraud.
The Challenges of Fraud Detection in the Insurance Sector
Because insurance fraud can take many different forms, it is challenging for insurers to identify and stop it. Some of the most prevalent types of insurance fraud include the following:
- Accidents staged: In this type of fraud, people create accidents on purpose in order to submit false insurance claims.
- False claims: People make false claims to obtain insurance payments for damages that did not happen.
- Identity theft: To submit false claims, fraudsters may use the identities of legitimate policyholders.
- Healthcare professionals commit medical billing fraud when they send insurance companies false invoices for medical services that were not provided.
The sheer volume and complexity of fraudulent claims make it challenging for insurers to spot and stop fraud using manual methods. This is where machine learning and artificial intelligence are useful.
AI and Machine Learning: A Method for Detecting Insurance Fraud
Massive amounts of data may be scanned by AI and ML systems, which can also identify trends that can point to fraudulent activity. By automating the fraud detection process, insurers can identify and stop fraud in real time, preventing billions of dollars in spurious claims.
Contrarily, it is challenging to implement AI and machine learning for fraud detection in the insurance sector. Among the principal difficulties are:
- The caliber of the data being examined has a significant impact on how accurate artificial intelligence and machine learning systems are. Insurance companies must make sure that their data is precise, comprehensive, and current in order to achieve the best results.
- Bias: AI and ML systems may bias towards certain assertions or people, resulting in erroneous or unfair findings. Insurance companies must ensure that their algorithms are impartial and fair in order to avoid bias.
- Privacy: Insurance fraud detection using AI and machine learning poses privacy concerns because sensitive personal information may be analyzed. To preserve customer privacy, insurers must follow data privacy rules.
Despite these drawbacks, implementing AI and machine learning for fraud detection in the insurance sector has several advantages. Among the numerous benefits are:
Faster and more accurate fraud detection: When compared to human methods, AI and machine learning algorithms are capable of evaluating enormous amounts of data in real-time. As a result, fraud can be identified and prevented more quickly and accurately.
By removing bogus claims, insurance firms can save billions of dollars in reimbursements and other expenses.
AI and ML algorithms help insurers detect fraud, handle valid claims, and improve customer service.
In the future, would AI still require human monitoring for fraud detection?
Despite all the advantages AI has for detecting fraud, it’s vital to keep in mind that these systems still need human oversight to be accurate and trustworthy, and they probably will in the future as well.
AI can detect fraud by scanning massive volumes of data and identifying suspicious patterns. AI can evolve to stay ahead of fraud tactics. What AI can accomplish on its own, though, still has some limitations.
The possibility of false positives and false negatives is one of the major constraints of AI in fraud detection. False positives happen when a valid transaction is marked as fraudulent, and false negatives happen when a valid transaction is not labeled as fraudulent. These mistakes might happen when the AI algorithms are not calibrated correctly or when they are based on data that is insufficient or wrong. Human monitoring is necessary to guarantee the accuracy and dependability of fraud detection systems.
AI algorithm development and calibration require human monitoring. Humans can verify data accuracy and completeness before training AI algorithms. They may also ensure the algorithms are calibrated, unbiased, and free of false positives and negatives. Human oversight is also needed to monitor fraud detection systems so organizations can spot and fix issues immediately.
AI-based fraud detection requires human oversight to investigate and resolve suspicious transactions. Even though AI may identify fraud tendencies and anomalies, humans must determine if these circumstances are fraudulent. Humans can detect and prevent fraud with knowledge and discretion that AI cannot.
In the end
With the development of AI and machine learning, insurers now have more tools at their disposal to identify and stop insurance fraud. By automating the fraud detection process, insurers can identify and stop fraudulent behavior in real time, preventing billions of dollars in phony claims.
Adopting AI and machine learning for fraud detection in the insurance sector is challenging, though, because of concerns about data bias, quality, and privacy. To get the best results and protect the interests of their clients, insurers must attempt to overcome these challenges.
Lastly, AI and ML have the ability to completely change how insurers identify and stop insurance fraud. By utilizing these technologies, insurers can detect fraud more quickly and accurately, reduce costs, and enhance the entire client experience.
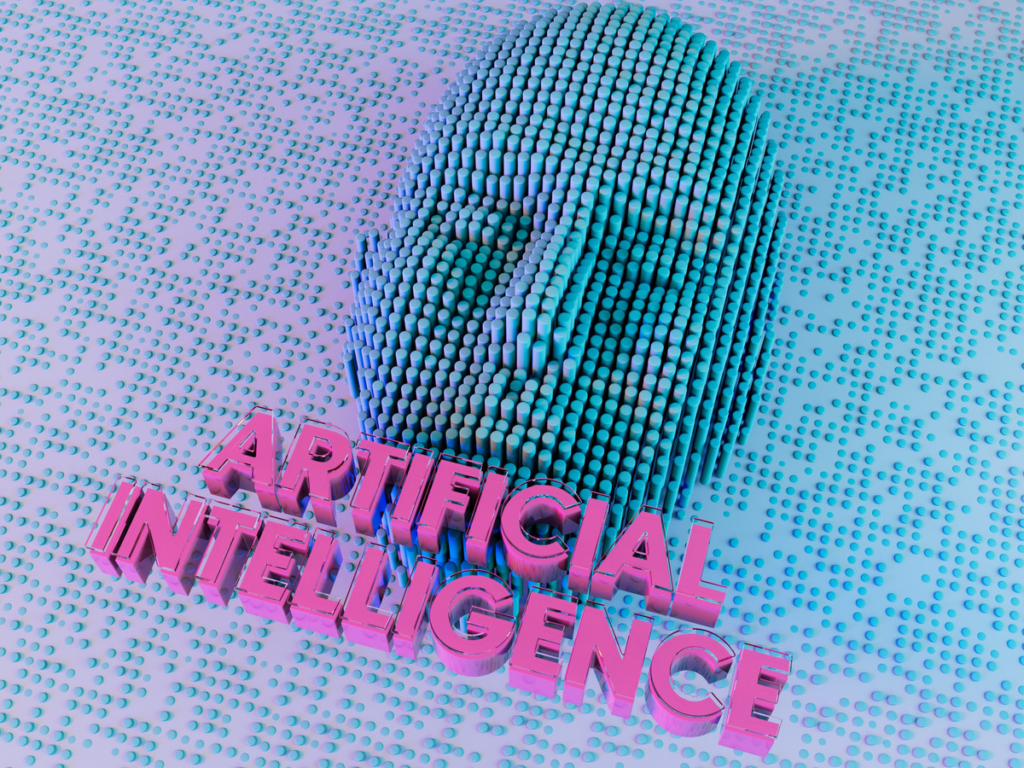