The new AI solution from Fuse helps lenders approve loans for gig workers without traditional credit and a single revenue source.
Due to a lack of traditional credit, self-employed and gig economy employees may find it difficult to get approved for loans. A new income verification tool has been created by Fuse, an AI-powered transaction analytics company, in an effort to support this industry and others that may find it difficult to demonstrate their creditworthiness.
Lenders can obtain probabilistic estimations and comprehensive information about potential borrowers’ revenue streams by using the Fuse tool. In spite of the absence of a single source of revenue, it nevertheless provides a more complete picture of the worker’s earnings. Because they don’t have a single source of income, this group has historically had difficulty getting lenders to verify and evaluate their credit. They ran the risk of being unjustly left out financially as a result.
The solution expands on the transaction enrichment features already present in Fuse. It offers thorough insight into an individual’s financial status through the application of cutting-edge machine learning and natural language processing. It gives lenders a probability-based approximation of a person’s income. Thus, even in the event of a variable salary, this empowers the lender to make an informed choice.
“Technology is transforming the financial sector for the better, facilitating the development of models that are more inclusive, safer, and built around the best interest of consumers,” says Sho Sugihara, CEO and co-founder of Fuse by Pave. The release of the Income Verification Tool is the next phase in our commitment to bringing about positive change. As a result, we pledge to improve loan availability and affordability for people with irregular revenue streams.
Substitutes for conventional credit
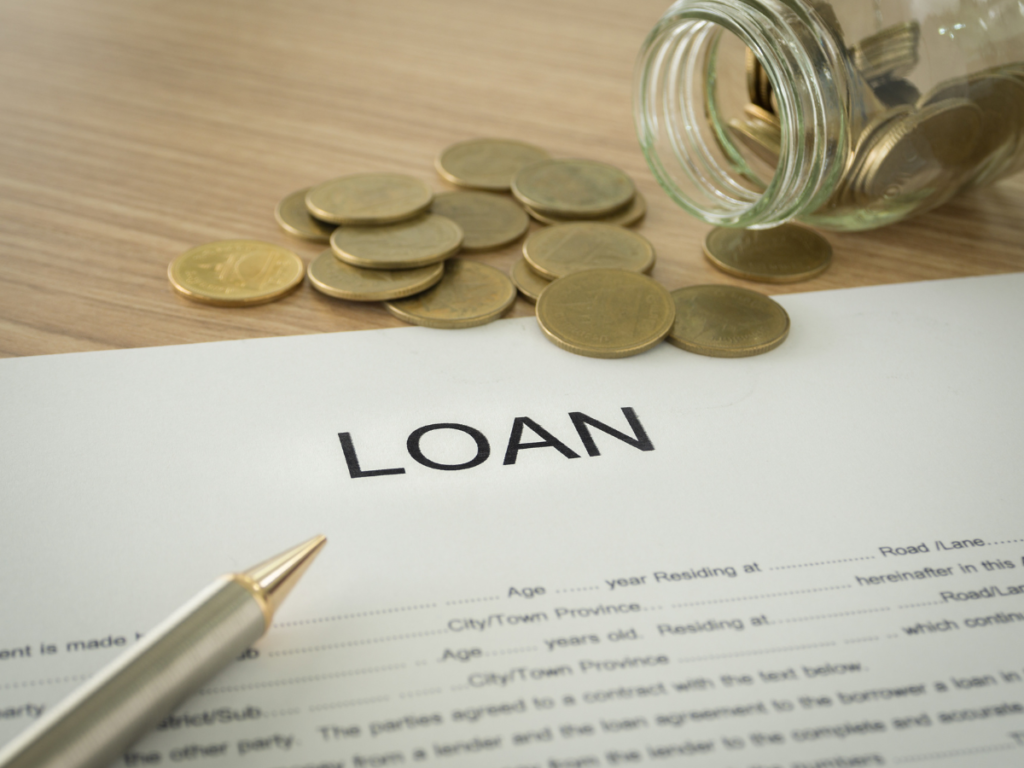
With the use of current transaction data, this information enables lenders to make quick, accurate, and responsible loan decisions. All of this is possible without depending only on the data related to the conventional credit evaluation procedure. Lenders are able to expand their clientele and enhance acceptance rates as a result. They can continue to be sure they are treating their clients fairly in the interim.
By utilizing technology to evaluate affordability in this manner, lenders can swiftly enter new, frequently underserved areas and provide lending alternatives in a responsible manner that promotes the borrower’s long-term financial stability.
Data from the subscription-based credit health builder software Pave is used to train the algorithm. It serves marginalized populations in the credit market, such as gig economy employees. It plays a major role in Fuse’s superior industry knowledge in lending to this industry. More than 20 banks’ worth of data are used by the tool. They all have distinct norms and standards for the formatting of transaction data.