Fintech expert Leon Gauhman explains why banking needs Large Language Models and Generative AI to counter the risks posed by Big Tech.
Don’t be deterred by the Chat GPT hype; banking needs large language models (LLMs) and generative artificial intelligence (AI) to combat the danger posed by Big Tech. According to fintech entrepreneur Leon Gauhman, CPO/CSO of digital product consultancy Elsewhen, which counts Spotify, Google, Microsoft, and Mastercard among its clients, this is the case.
In light of the fact that neobanks’ apps outperform legacy banks’ and that Apple and Google are gaining ground in the battle for supremacy in the digital wallet market, Gauham contends that adopting LLM will enable banking incumbents to tip the scales back in their favor.
The metaverse was the overhyped focus of tech talk in 2022; this year, OpenAI’s ChatGPT is its replacement. The all-knowing chatbot appeared to supplant professors, journalists, and attorneys in the frenzy.
Yet, ChatGPT’s flaws show that issues must be fixed before these technologies can fulfill their revolutionary potential.
But, incumbents and neobanks should continue to pay attention since ChatGPT and kindred generative AI tools’ most essential lesson is the promise of large language models (LLMs), their foundational technology. Microsoft invested $10 billion in OpenAI, the originator of ChatGPT, while Google and Microsoft released LLM-powered business communication products.
The pace with which big tech, an up-and-coming rival to incumbents and neobanks, is implementing LLMs into its operations, highlights the significance big tech places on this technology.
New advancements in AI-driven deep learning may allow incumbents and neobanks to tip the scales in their favor in the following four sectors, where Apple and Google are leading the race for supremacy in the digital wallet market:
1.Clients may get access to a personal AI banker
Large language models contribute to one of banking’s greatest strengths: its exclusive databases. Instead of attempting to imitate their competitors with piggyback products, banks may use large pools of customer data and insights to employ deep learning and natural language processing capabilities to generate their own valuable IP.
A well-trained ChatGPT version might create a personalized AI banker that gives clients real-time suggestions tailored to their needs. By creating space for genuinely innovative, responsive products that meet user expectations, this may instantly alter banking’s reputation for online user experiences.
2.The use of AI can increase banking productivity
Big language models improve client experience and staff productivity by increasing resources. Microsoft claims its Copilot tool will help Office users prepare presentations and meetings with relevant updates. Google calls the AI tool a “collaborative partner” that recommends, summarizes, and provides insights.
In general, these technologies have the ability to completely transform labor-intensive workflows for procedures like KYC, compliance, and AML. The budgetary, productivity, and time implications are enormous because these fundamental processes make up 15-20% of bank budgets.
3.The keys to a top-gear digital transformation are large language models
Legacy players have failed to support digital banking, but LLMs may rejuvenate the entire financial services stack. New vendors will create substantial open-source models to train LLMs using bank data. So, banks can use generative AI in product design, mobile banking, cybersecurity, and employee onboarding.
For instance, Swedbank already employs generative adversarial networks to spot fraudulent transactions, using synthetic modeling to comprehend and foresee irregularities that go unnoticed. This ability turns into a superpower in the drive to transform banking into a digital industry, allowing banks to outwit more agile rivals.
4.A new era of proprietary modeling is ushered in by large language models
LLMs give banks the ability to take private data, mine it for insightful information, and use the resulting actionable data to create conversational user interfaces or new personal banking/wealth management techniques.
For instance, LLMs could examine ten years’ worth of banking data on mortgage defaults and utilize the results to develop a fresh, adaptive underwriting framework for improved lending judgment.
Last reflection
Banks are sometimes criticized for being overly conservative and slow to react in these days of hyper-innovation. An attentive approach is preferable while dealing with LLMs. AI models have a tendency to “hallucinate,” producing biases or mistakes, as we have seen with Bing ChatGPT-powered search and Google’s AI chatbot Bard. It is generally benign for an AI chatbot to mistakenly respond incorrectly about NASA’s James Webb Space Telescope.
Contrarily, an AI chatbot for a bank brand giving false financial advise would be extremely bad news. A rigorous human review and input are necessary, not optional, during the data training stages because of this potential risk.
Yet being cautious does not justify inaction. Banks must continue to advance their knowledge of, experimentation with, and training of LLMs if they are to future-proof their operations against the danger of big tech. The biggest risk for banks is doing nothing, as past recent technological shifts have shown. It’s time to make up ground by transforming LLM into a key business metric that prioritizes customer satisfaction.
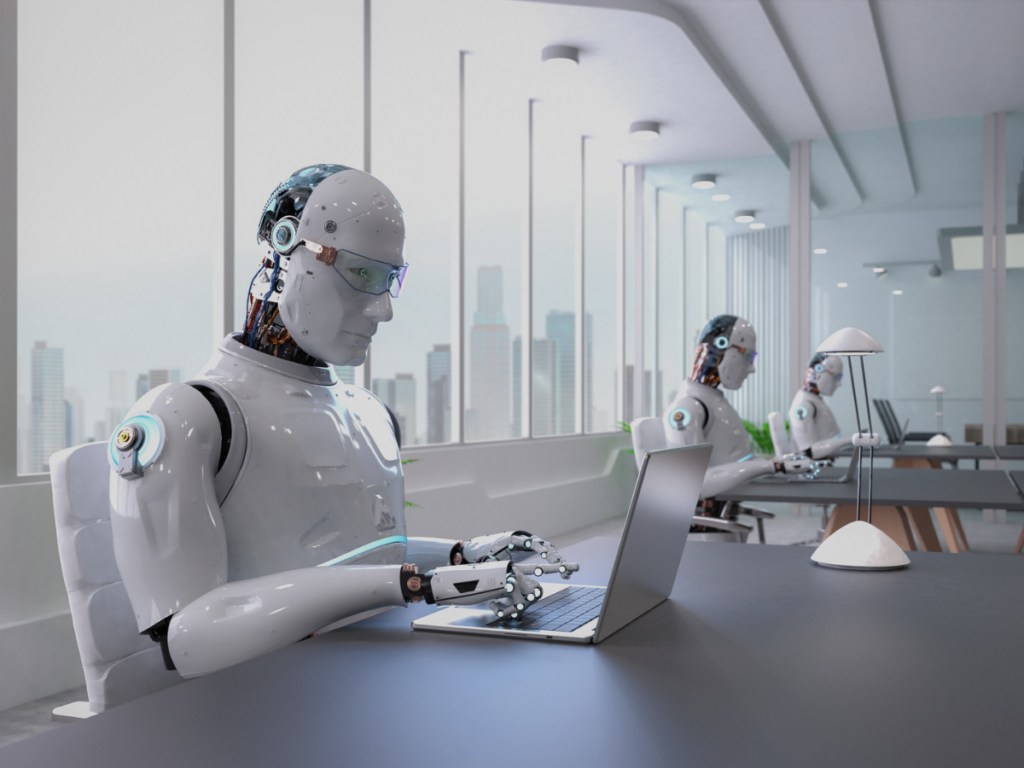